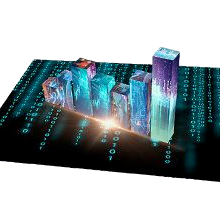
Typical feature reduction algorithms suffer from problems relating to class of features (categorical, continuous) and the complexity resulting from the number of features, thus making redundant feature elimination a difficult task. Additionally, it is not clear as to how the objective function affects the feature selection.
In this hack session, I will discuss about how to use moving set of thresholds for feature selection, which are optimized based on a Markov Chain Monte Carlo that adjusts the thresholds based on the final model fitness.
As part of the talk, I will give a live demonstration of this approach in action. The method will be contrasted against commonly used methods such as forward selection, vanilla ML algorithms, and correlation based cut-offs to highlight its usefulness. The notebook used for the demonstration can be made available to participants.
Finally I’d like to mention that the motivation for this talk comes from my experience conversing with data scientists on feature selection. Many of them do not have a consistent method by which they eliminate a large number of redundant or noisy features. This method helps close that gap.
HACKERS
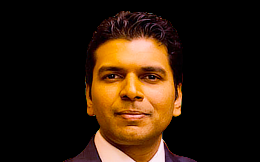
Sidharth Kumar
Sidharth heads the decision science team at Flipkart – a team focused on solving machine learning intensive – high business impact problems. He and his team tackle some of the most challenging ML problems encountered at Flipkart including pricing, selection, supply chain optimization, and customer engagement modeling.
Sidharth has a PhD in Astrophysics from the University of Maryland, College Park and a B.Tech from IIT Madras. Prior to joining Flipkart, he worked as an associate strategist in Goldman Sachs, and as a machine learning quantitative researcher at a hedge-fund, ACR capital in New York. He has published in several journals and presented at several conferences all over the world.
Duration of Hack-Session: 1 hour