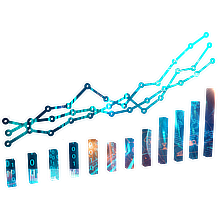
Real world processes and businesses keeps changing, with one moving part changing another over time. Can we capture these changing relationships? Can we use multiple variables to find risks on key interesting ones? We will take a journey culminating in the most recent developments in the field. What methods work well and which break? What can we use in practice?
Structure of the hack Session:
- Business Problem Definition
- Solutions that do not work well (ARIMA, ARIMAX etc.)
- Finding Relationships
- Temporal Causal Models – Technical Algorithm and Hack Demo
- Dealing with Non-Stationarity
- Finding change in distribution: Kullback-Leibler Divergence
- Windowing, Weighting techniques
- LSTM – Technical Algorithm and Hack Demo
- What else can we do to understand time series? Wavelets, Fourier Transformations
Key Takeaways from the Talk:
At the end of the hack session, you will be able to- Explore new techniques for finding causal relationships between time series and use multiple variables to forecast others.
- Understand that we need not lose details of the process inherent in the data in the process of converting non-stationary time series to stationary.
Hackers
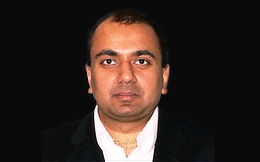
Vikas Agrawal
Vikas Agrawal works as a Senior Principal Data Scientist in Cognitive Computing for Oracle Analytics Cloud. His current interests are in automated discovery, adaptive anomaly detection in streaming data, intelligent context-aware systems, and explaining black-box model predictions.
Vikas received a B.Tech. in Electrical Engineering from the Indian Institute of Technology, New Delhi (1997), an MS in Computer Science and a PhD in Computational Modeling from University of Delaware, with post-doctoral research at California Institute of Technology (CalTech, Pasadena, CA) with colleagues from NASA’s Jet Propulsion Labs (JPL) for NSF’s FIBR.
Duration of Hack-Session: 1 hour